Table Of Content
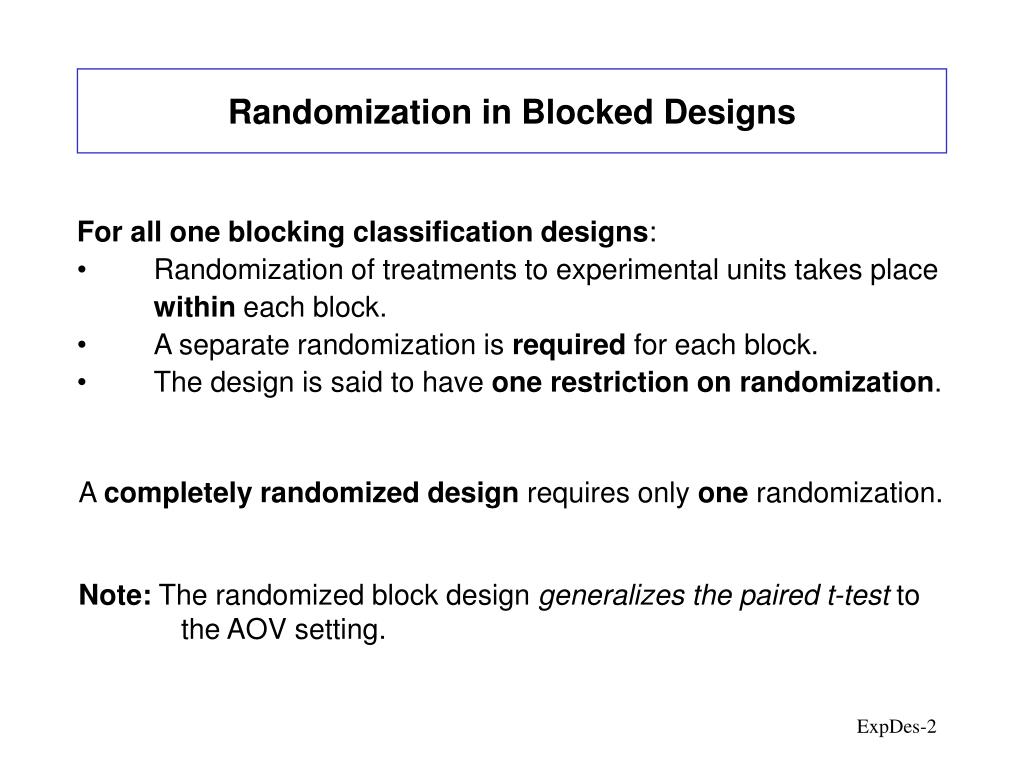
The first 3 indicates the number of rows we want in the matrix; since we have 3 populations in each block, we need our matrix to have 3 rows. The first step in using the RCBD is to recognize the source(s) of potential heterogeneity among plots (experimental units). In field research, this potential most often exists between plots situated on different soil map units, for example, as the slope changes.
Estimate Effects Using ANOVA
This means that the first experimental unit will get treatment \(B\), thesecond \(D\), and so on. This assignment can then be used to apply the treatment levels appropriately to pots on the greenhouse bench. We need to be able to randomly assign each of the treatment levels to 6 potted plants. To do this, assign physical position numbers on the bench for placing the pots.
Associated Data
Following Equations 10 and 13, ICCC can be estimated on the basis of a two-level logistic regression analysis using the intervention group data, where compliance type is observed. It is shown that the impact of ICCC on the coverage rate increases as the distance between complier and noncomplier means increases. Depending on how heterogeneous compliers and noncompliers are, their outcomes may have a substantial distance in the absence of intervention treatments. Whether this distance is large enough (e.g., 0.5–1.0 SDs or larger) to connect ICCC with a substantial bias in variance estimation cannot be observed from the data since compliance types are not known among individuals assigned to the control condition. However, if it is reasonable to assume the exclusion restriction and monotonicity, complier and noncomplier means can be estimated using the standard CACE estimation method. If this distance is substantial and ICCC is moderate to high, one should be aware that not only ICCY but also ICCC will contribute to variance misestimation, necessitating simultaneous consideration of clustering and noncompliance in the analysis.
Simultaneous Consideration of Clustering and Noncompliance
The M step computes the complete-data ML estimates with complete-data sufficient statistics replaced by their estimates from the E step. In the current study, ML-EM estimation of CACE has been carried out by Mplus Version 4.2 (L. K. Muthén & Muthén, 1998–2007). In the design of experiments, completely randomized designs are for studying the effects of one primary factor without the need to take other nuisance variables into account. This article describes completely randomized designs that have one primary factor. The experiment compares the values of a response variable based on the different levels of that primary factor.
Impact of Outcome Intraclass Correlations
On the basis of a simple two-level analysis using only the intervention group data, where compliance type is observed, ICCYn (from a noncomplier-only analysis) and ICCYc (from a complier-only analysis) can be estimated. Similarly, ICCC can be estimated on the basis of a two-level logistic regression analysis using only the intervention group data. If all these estimates are very small, there is less need to employ the two-level CACE approach. As discussed earlier, depending on how heterogeneous complier and noncompliers are, their outcomes may have a substantial distance in the absence of intervention treatments.
The coverage of the CACE estimate is somewhat lower when the correlation between εnbj and εcbj is substantial (e.g., 0.5) than when the correlation is zero. However, both settings lead to similar results as complier and noncomplier means have a substantial distance (i.e., 1.0 SD apart). In the potential outcomes approach, identification of causal effects (including ITT effect) is built on the basis of two critical assumptions. Second, it is assumed that each individual’s potential outcomes are uncorrelated with other individuals’ treatment assignment status, which has been labeled as stable unit treatment value (SUTVA; Rubin, 1978, 1980, 1990).
Differentiating CRD from other research design methods
Therefore, a coverage rate below 0.95 mainly indicates that standard error estimates do not appropriately reflect variance inflation due to clustering of compliance behavior. In other words, the level of significance of the treatment effect is overstated. When compliers and noncompliers have homogeneous outcome distributions (0.0 SDs apart), the coverage rate stays close to the nominal level irrespective of the change in ICCC. When complier and noncomplier outcome means are 0.5 SDs apart, the coverage rate begins to be affected especially by high ICCCs such as 0.8 and 1.0. When complier and noncomplier outcome means are 1.0 SD apart, the coverage rate decreases substantially with moderate to high ICCCs.
11: Randomized Complete Block Design
Note that whenever we transform the response, this comes at the price of a newinterpretation of the parameters! Care has to be taken if one wants to interpretstatistical inference (e.g., confidence intervals) on the original scale as manyaspects unfortunately do not easily “transform back”! In addition, as with any other statistical test, power increases with sample size.Hence, for large samples we get small p-values even for very small deviationsfrom the model assumptions.
Cluster Randomized Trials With Treatment Noncompliance
A comparison of clinical development pathways to advance tuberculosis regimen development - BMC Infectious ... - BMC Infectious Diseases
A comparison of clinical development pathways to advance tuberculosis regimen development - BMC Infectious ....
Posted: Fri, 09 Dec 2022 08:00:00 GMT [source]
Therefore, analyses ignoring the connection between compliance and clustering may not provide valid results. Although randomized field experiments often suffer from both noncompliance and clustering of the data, these features have been studied as separate rather than concurrent problems. On the basis of Monte Carlo simulations, this study demonstrated how clustering and noncompliance may affect statistical inferences and how these two complications can be accounted for simultaneously. In particular, the effect of the intervention on individuals who not only were assigned to active intervention but also abided by this intervention assignment (complier average causal effect) was the focus. For estimation of intervention effects considering noncompliance and data clustering, an ML-EM estimation method was employed.
Use of Sequential Multiple Assignment Randomized Trials (SMARTs) in oncology: systematic review of published ... - Nature.com
Use of Sequential Multiple Assignment Randomized Trials (SMARTs) in oncology: systematic review of published ....
Posted: Mon, 26 Dec 2022 08:00:00 GMT [source]
However, these random effect between-cluster parameters show somewhat low coverage rates. The results imply that, unless large numbers of clusters are available, the level of significance of between-cluster random effects should be interpreted with caution. We expect that the quality of standard error estimates will improve as the number of clusters increases (the number of clusters is currently 50 per compliance type). Finally, in the logistic regression of compliance, between-cluster variation of compliance is captured by the between-cluster residual variance (ψb2), indicating a sizable ICCC given covariates. In particular, the impact of ICCC is quite striking, implying the need for checking ICCC when CRTs are accompanied by treatment noncompliance.
While other designs might offer more control and complexity, the fundamental simplicity of CRD will continue to hold significant value in the rapidly evolving research landscape. A CRD experiment involves meticulous planning and execution, outlined in the following structured steps. The idea of using additional covariates is very general and basically applicableto nearly all the models that we learn about in the following chapters. In that sense, the results of a power analysis are typically not very precise.However, they should still give us a rough idea about the required sample sizein the sense of whether we need 6 or 60 observations per group. A nice side effect of doing a power analysis is that you actually do the wholedata analysis on simulated data and you immediately see whether this works asintended.
For example, to discern the impact of varying temperatures on the strength of a metal alloy, engineers might utilize CRD. In this scenario, the different temperatures represent the single factor, and the alloy samples are randomly allocated to be tested at each designated temperature. This random assignment minimizes the influence of extraneous variables, ensuring that the observed effects on alloy strength are primarily attributable to the temperature variations. Agricultural research was among the earliest fields to adopt the use of Completely Randomized Design. The broad application of CRD within agriculture not only encompasses crop improvement but also the systematic analysis of various fertilizers, pesticides, and cropping techniques.
This improves the precision of the F-test for population because the Error MS decreased as a result of partitioning some of the variation into blocks. Since the Error MS is smaller for the RCBD, there will also be improved precision for comparing means because the standard errors used to calculate these tests are calculated from the Error MS. We trust the results more if these differences are consistent across blocks (left-hand side). When the B x T interaction is large, (right-hand side), the random error obscures the treatment differences. Suppose that soybean yield means for three herbicide treatments are 2.7 t/ha for herbicide A, 3.0 for B, and 3.3 for C.
They are predefined algorithms that help us in analyzing the differences among group means in an experimental unit. For instance, a manufacturer keen on minimizing product defects may deploy CRD to empirically assess the effectiveness of various inspection techniques. In industrial engineering, Completely Randomized Design plays a significant role in process and product testing, offering a reliable structure for the evaluation and improvement of industrial systems. Engineers often employ CRD in single-factor experiments to analyze the effects of a particular factor on a certain outcome, enhancing the precision and objectivity of the assessment. While CRD's simplicity and flexibility make it a popular choice for many research scenarios, the optimal design depends on the specific needs, objectives, and contexts of the study. Researchers must carefully consider these factors to select the most suitable research design method.
However, the results of these analyses are not only hard to interpret as causal effects but also subject to substantial bias (Robins & Greenland, 1994; Sheiner & Rubin, 1995). This study employs the CACE estimation method, which is considered a better alternative to as-treated or per-protocol analysis. The key advantage of the CACE estimation method is that causal effect is defined on the basis of individuals’ potential outcomes under every treatment assignment status (Angrist et al., 1996; Frangakis & Rubin, 2002).
No comments:
Post a Comment